Program
Thursday 6th
9.15 |
Registration
|
10.15 |
Opening
|
10.30 |
Keynote speaker – Jan Peters
|
11.30 |
Hair
|
|
|
12.20 |
Lunch
|
13.40 |
Deformable bodies
|
|
|
15.20 |
Coffee break
|
15.50 |
Fluids
|
|
|
19.00 |
Conference dinner
|
Friday 7th
9.15 |
Keynote speaker – Stéphane Cotin
|
10.15 |
Coffee break
|
10.45 |
Motion synthesis
|
|
|
12.00 |
Lunch
|
13.30 |
Medical simulation and VR
|
|
|
14.45 |
Coffee break
|
15.15 |
Motion synthesis and motion planning
|
|
|
16.05 |
Closing
|
Invited talks
Jan Peters
Title:
Machine Learning of Motor Skills for Robotics
Abstract:
Autonomous robots that can assist humans in situations of daily life have been a long standing vision of robotics, artificial intelligence, and cognitive sciences. A first step towards this goal is to create robots that can learn tasks triggered by environmental context or higher level instruction. However, learning techniques have yet to live up to this promise as only few methods manage to scale to high-dimensional manipulator or humanoid robots. In this talk, we investigate a general framework suitable for learning motor skills in robotics which is based on the principles behind many analytical robotics approaches. It involves generating a representation of motor skills by parameterized motor primitive policies acting as building blocks of movement generation, and a learned task execution module that transforms these movements into motor commands. We discuss learning on three different levels of abstraction, i.e., learning for accurate control is needed to execute, learning of motor primitives is needed to acquire simple movements, and learning of the task-dependent “hyperparameters” of these motor primitives allows learning complex tasks. We discuss task-appropriate learning approaches for imitation learning, model learning and reinforcement learning for robots with many degrees of freedom. Empirical evaluations on a several robot systems illustrate the effectiveness and applicability to learning control on an anthropomorphic robot arm. These robot motor skills range from toy examples (e.g., paddling a ball, ball-in-a-cup) to playing robot table tennis against a human being.
Biography:
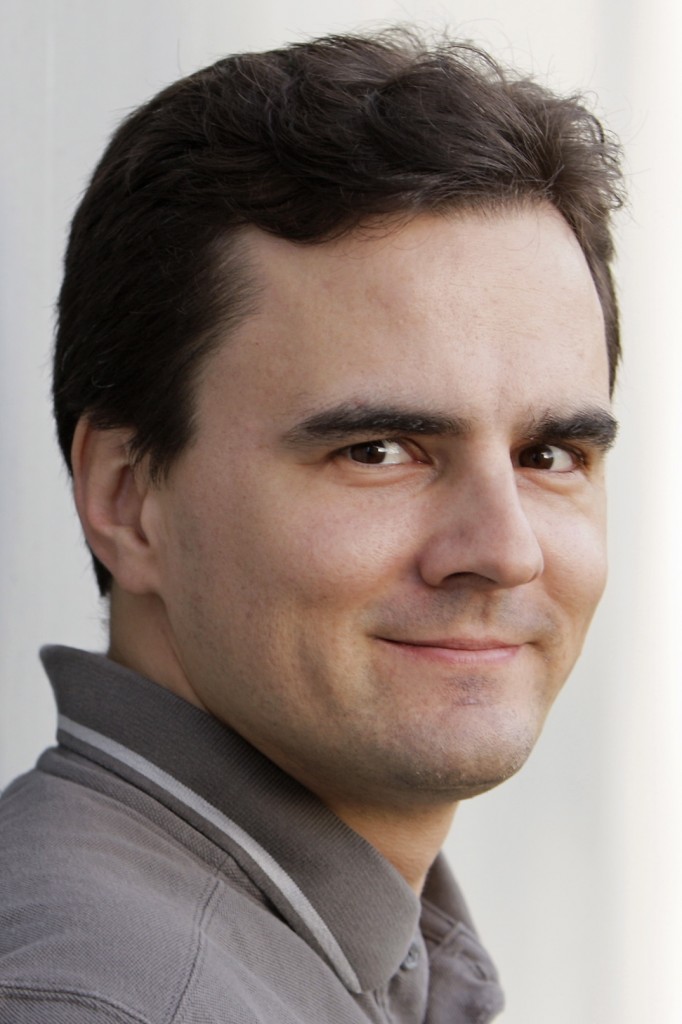
Jan Peters
Jan Peters is a full professor at the Technische Universitaet Darmstadt and a senior research scientist at the Max Planck Institute for Intelligent Systems (MPI-IS) heading the interdepartmental robot learning group. Until 2011, he was was senior research scientist at the Dept. for Empirical Inference and Machine Learning of the Max Planck Institute for Biological Cybernetics (MPI-KYB) in Tuebingen, Germany. He graduated from University of Southern California (USC) with a Ph.D. in Computer Science. His Ph.D. Thesis received the Dick Volz Best 2007 U.S. Ph.D. Thesis Runner-Up Award in 2011 based upon quality and post-defense impact. He has completed masters degrees in Electrical Engineering (Dipl.-Ing./TU Muenchen), Informatics (Dipl-Inform./FernUni Hagen), Computer Science (M.Sc./USC) and Mechanical Engineering (M.Sc./USC). Jan Peters has been a visiting researcher at the Department of Robotics at the German Aerospace Research Center (DLR) in Oberpfaffenhofen, Germany, at Siemens Advanced Engineering (SAE) in Singapore, at the National University of Singapore (NUS), and at the Department of Humanoid Robotics and Computational Neuroscience at the Advanded Telecommunication Research (ATR) Center in Kyoto, Japan. Jan Peters has been invited for the prestigious Early Career Spotlight Plenary at Robotics: Science & Systems as well as the Frontiers of Artificial Intelligence talk at the European Conference on Artificial Intelligence. His research interests include robotics, nonlinear control, machine learning, reinforcement learning, and motor skill learning.
Stéphane Cotin
Title:
Numerical Simulation in Medicine: From Training to Computer Assisted Interventions
Abstract:
The variety and complexity of Medicine, as well as its ethical importance in today’s society, have been a strong motivation in many scientific and technical disciplines. While medical image processing is today an integral part of modern medicine, new fields are emerging, such as robotics, simulation, augmented reality, or workflow analysis. In this talk I will highlight the increasingly important role of (real-time) numerical simulation in various domains, such as training, but also for planning and in the assistance of complex interventions. I will illustrate the impact of simulation through a series of results in various areas of medicine, such as interventional radiology, ophthalmology, and laparoscopic surgery. Finally I will discuss some of the remaining challenges that need to be met to further accelerate the introduction of simulation in medicine.
Biography:
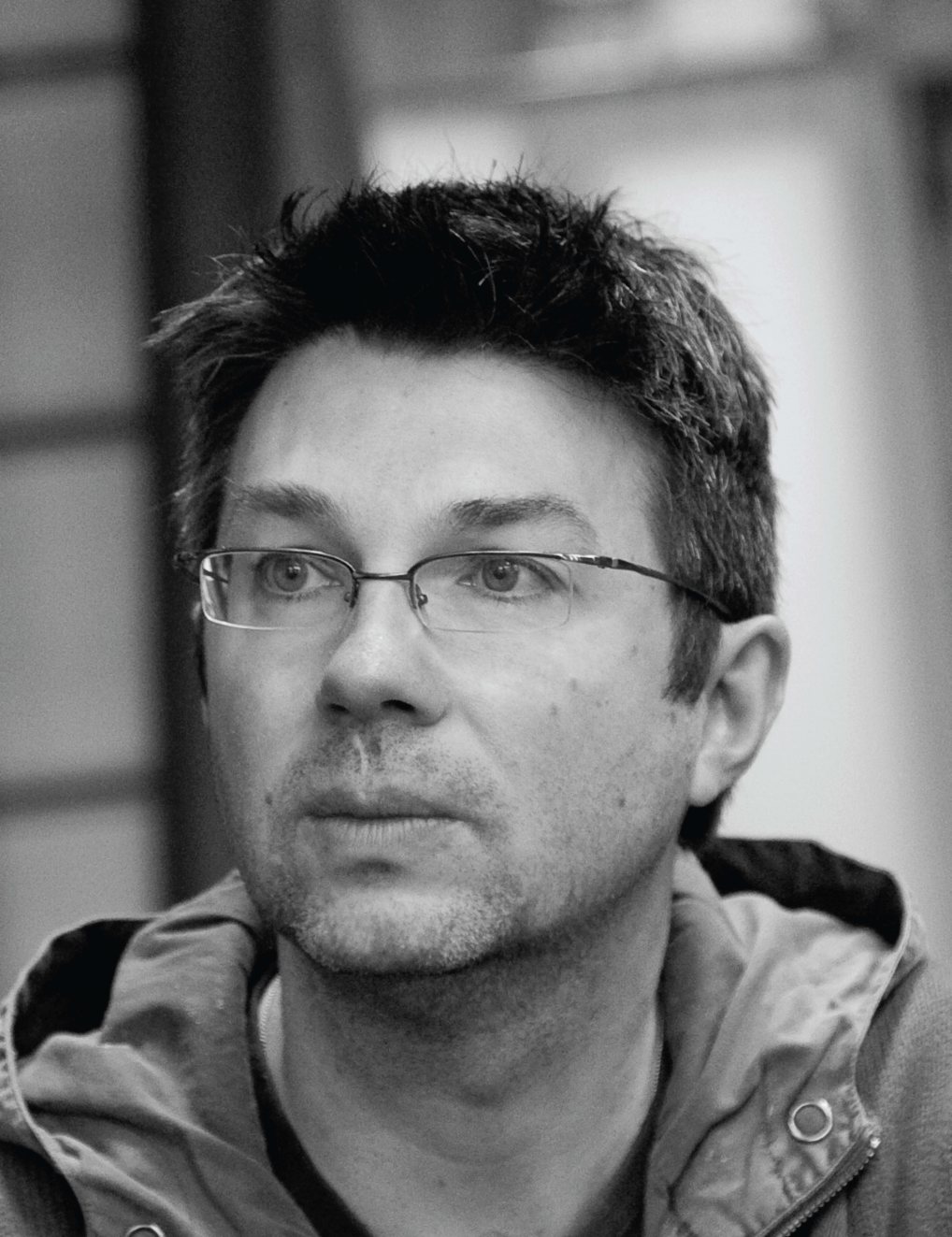
Stéphane Cotin
Stéphane Cotin is the scientific leader of the SHACRA team at Inria Lille. Stephane joined Inria in 2007 to hold a position of Research Director, and since 2012 he is highly involved in a new institute (IHU) based in Strasbourg and dedicated to new, hybrid, surgical techniques. Both the Lille and Strasbourg groups share the same scientific objectives, essentially related to interactive medical simulation, for training and planning purposes. Stephane’s personal research interests include biomechanical modeling of soft tissue, mechanical modeling of flexible medical devices, patient-specific anatomical modeling adapted to real-time simulation, augmented reality, image-guided simulation, and performance metrics for training systems. Stephane is also responsible for the development of a national initiative on Medical Simulation using the SOFA framework (www.sofa-framework.org) as a common platform for research, integration and validation of new algorithms. Before joining INRIA, Stephane was Instructor at Harvard Medical School and Research Lead for the Medical Simulation Group at CIMIT in Boston where he was responsible for defining research directions and technical infrastructures for several simulation projects. These projects included a Chest Trauma Training System, a Computer-Enhanced Laparoscopic Training System and an Interventional Radiology Training System. Stephane is author or co-author of about 75 scientific articles.